By Andy Freeberg
ME Assistant Professor Krithika Manohar developed an approach known as PIXel Identification Despite Uncertainty in Sensor Technology, or PIXI-DUST, to improve Boeing’s process for assembling airplane wings. Photo courtesy of The Boeing Company with inset from Manohar et al., Journal of Manufacturing Systems 2018
Machine learning presents tremendous opportunities to improve how we build airplanes. ME Assistant Professor Krithika Manohar is just getting started.
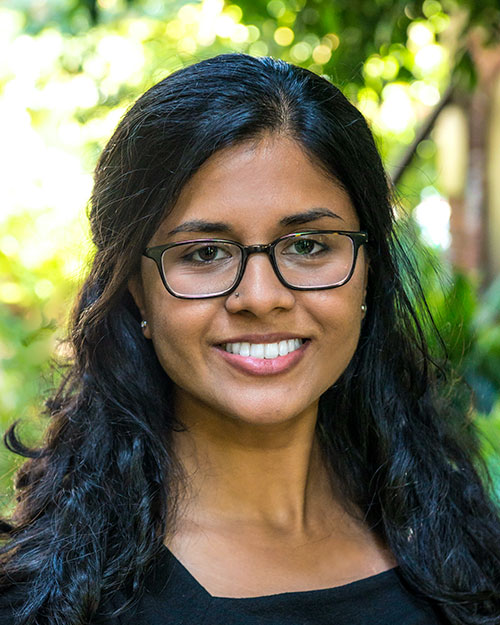
Krithika Manohar, assistant professor of mechanical engineering
Krithika Manohar is one of ME’s newest faculty members, having joined as an assistant professor in January 2021, but she’s no stranger to the department. Manohar received her Ph.D. in Applied Mathematics from the UW in 2018 and did her doctoral research through the Boeing Advanced Research Center (BARC), based out of ME.
An expert in the kinds of math that allow engineers to predict and control what’s going on inside complex and dynamic systems, Manohar’s skills are in high demand. She describes machine learning as “nothing but optimizing over data,” and in the sprawling and complex modern process of airplane manufacturing, she sees many prospects for these emerging approaches to make a big impact.
How did you get interested in machine learning and mechanical engineering?
I actually spent my first two years out of high school studying animation at the Massachusetts College of Art. While I was there I was required to take math classes, and after I took an introductory calculus course, I was hooked. I switched to a mathematics and computer science double major. I started doing research working with professors on projects about fractals, parallel computing and other topics I was fascinated with.
When I decided to do a Ph.D., my choices were between math and computer science. Ultimately, I was drawn to applied math because it bridges the two fields. That decision led me to the UW, where I got involved in BARC work with ME Professor Steve Brunton and applied math Professor Nathan Kutz.
I’ve been fascinated by airplanes since I was young. In first grade, I did a project on flight; I remember how fun it was to construct little paper and wood airplanes and test them out. When I started working with BARC I got to tour the Boeing plant in Everett. Seeing the scale and complexity of constructing real planes was mind-blowing.
Related story
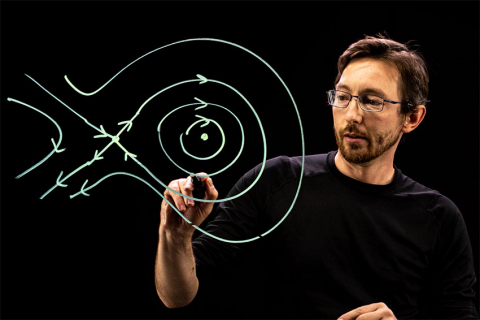
research center
AI Institute for Dynamic Systems
UW leading new NSF institute for using artificial intelligence to understand dynamic systems.
Your doctoral research has had a big impact for Boeing. Can you explain what you did?
All parts of an aircraft are manufactured separately from each other at different facilities around the world. They obey strict tolerances and high standards of quality control and at the end when they’re joined together, any remaining gaps and variations are shimmed to maximize the structural performance.
I was specifically studying the connections in the wing and where the wing attaches to the body, or fuselage, of the plane. The process used to be done manually — Boeing would put the wing and fuselage together, carefully measure each gap, and then take it all back apart and manufacture the necessary shims. That process was labor-intensive, so more recently Boeing moved to robotic laser scans. They gather billions of data points to get a precise measurement of all the parts and do a computationally demanding alignment to determine what shims are needed.
The goal of my Ph.D. research was to find out if you could measure in optimized and specific places to predict where the gaps would be. In this case the machine learning approach works especially well because the parts go through such a stringent manufacturing process, which allows you to extract the most prevalent patterns and optimize with a lot of reliability.
In the end our algorithm successfully predicted 99% of shim gaps using only around 3% of the typical measurements. Boeing thoroughly validated the new process, and it now saves them a great deal of time and computational resources.
A cartoon illustrates advancements to shimming, a key step in assembling an airplane. Image courtesy of The Boeing Company from Brunton et al., American Institute of Aeronautics and Astronautics Journal 2021
And you named your approach PIXI-DUST?
Haha, yes, it’s known within Boeing as PIXel Identification Despite Uncertainty in Sensor Technology, or PIXI-DUST. We were at a meeting and one of the lead Boeing researchers said it was “like scattering pixie dust on the wing to predict 99% of the places where you need to shim.”
Is it challenging to apply machine learning to an area like air travel?
I like to describe machine learning as optimizing over data. There are a lot of ways to do it, so when you’re using it in areas where there are strict margins for error, like in aerospace and other safety-critical applications, it’s possible to enforce constraints and be sure your results are very reliable and robust. There’s an emerging research area focused on machine learning with guarantees, and it’s a topic I’m very interested in.
The other critical factor is redundancy. This is a mathematical question too — if you’re optimizing sensor placement, you need to build backup sensors into your algorithm that will fill in if a sensor fails.
In simulations you’re able to work with almost infinite amounts of data, but a real airplane can only get data from a limited set of sensors. Machine learning can help bridge that gap so that airplanes are engineered to optimize sensor placement and detect sensor failure.
Looking ahead, what excites you most about this research?
I’m part of the new AI Institute for Dynamic Systems that was recently announced by the National Science Foundation and topics like sensor optimization and machine learning with guarantees are things I’m excited to investigate further. When you think about turbulence and all the wind swirling around an aircraft at every moment, you can appreciate how hard it is to predict what will happen in every possible circumstance. As we get better at incorporating known physics into our models, we’re going to be able to improve how well we can predict failures, incorporate safety constraints and solve complex engineering problems that fit well into mathematical and data approaches.
Beyond airplanes, we’re exploring how machine learning can improve some of Boeing’s other manufacturing processes, for example, helping the company figure out how to standardize brackets and other commonly needed parts. Ergonomic safety is an area we’ve worked with Amazon on in the past, and we’re now working with Boeing on that, too.
As a student who studied these problems and now as an assistant professor, I see so many opportunities in our partnership with Boeing. Engineers at Boeing have a chance to learn these state-of-the-art techniques, while students and faculty at the UW get to collaborate with real-world engineers and see tangible impact from our work. It’s a very beneficial relationship both ways.
Find out more
Read the recent UW news release announcing the AI Institute for Dynamic Systems or visit their website.
Visit the Boeing Advanced Research Center to learn about R&D partnerships between the UW and the Boeing Company.
Explore ME research in Data Science & Machine Learning.
Originally published October 20, 2021