By Chelsea Yates
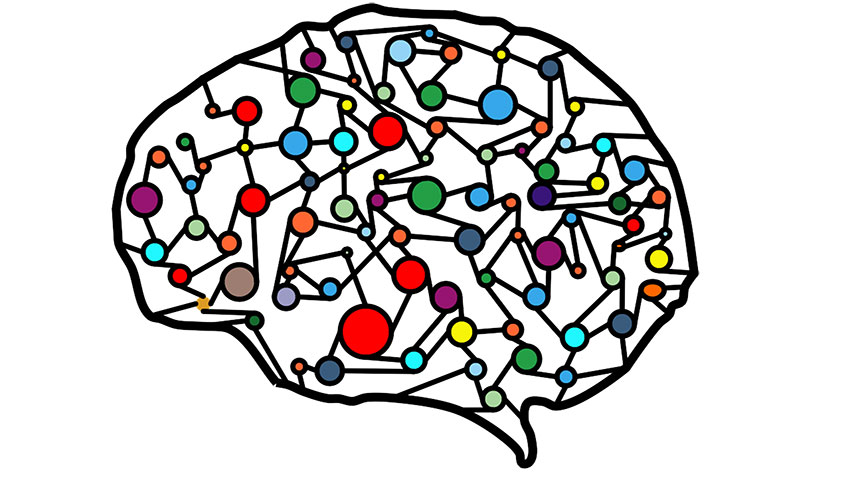
Data science offers a new frontier of exploration, and with decades of experience in systems, sensors, controls and fluids research, mechanical engineers are helping to shape the field’s future.
ME associate professor Steve Brunton is one of these leading researchers. Currently a data science fellow at the UW’s eScience Institute, he is involved in cutting-edge work that applies data science, machine learning and dynamical systems to classical engineering problems in fluid mechanics and airplane design. We recently sat down with Brunton to examine data science through a mechanical engineering lens and discuss his research, including an unexpected creature at the center of it: the moth.
How are data science and big data related?
Data science is an umbrella term for acquiring, managing, cleaning, formatting, storing and interpreting data. Data scientists extract information and reveal patterns. Science has always been driven by data; however, data science has become more popular due in part to the rapid growth of “big data” — complex data too large to be easily stored or analyzed in traditional systems. It’s all around us; sources include email, social media posts, digital media files, mobile phones, medical records, financial records and increasingly engineering systems, such as aircraft and factories. We use data science as a tool to manage and assess it.
How so?
Mostly through machine learning and data analysis. Once a complex data set is collected, we apply machine learning, which refers to the process of building models to manage and describe data and automate its analysis. We develop and use algorithms to identify patterns and insights. Then we extract information, form conclusions and consider future applications.
Why are mechanical engineers important to data science?
Machine learning relies on computer science, statistics and applied mathematics. Because of their diverse background, mechanical engineers work effectively across these areas. The future of machine learning — and data science — will involve a deeper connection with physics; the more we want to apply machine learning to real-world problems, the more we need people who know how to work with nonlinear and dynamical systems, who can work in chaotic environments with ever-changing factors. For example, how do we account for turbulence in airplane design? How do we better manage the impact of injury in a human body? How do we collect and convert energy from dynamic sources like the sun, wind and waves? Mechanical engineers have long been working in such areas; because of our experience with systems, sensors, controls and fluids, we have much to offer.
How did you become interested in data science?
My background is in fluid mechanics and dynamics, and I’m particularly interested in deepening the relationship between physics and machine learning. There has been a bit of a divide between the computer science and statistics elements of machine learning, but as the technology grows, so does the need to unite them. Especially if we want to apply machine learning to systems that aren’t linear, clean or predictable — most real-world systems. To advance machine learning, we will need a “new physics” for such systems, and that’s exciting.
Currently, we can look to nature to know that this is possible. Biological systems often don’t operate under the assumptions required for big data or deep learning; instead they work with limited and incomplete data. For example, a moth flies without velocity field measurements, a Ph.D. in fluid mechanics or knowledge of governing equations. Instead, it relies on a few well-placed sensors and a robust neural control system. This system provides proof-by-existence in advanced capabilities based on limited data.
You mentioned a moth – how has it informed your research?
I explore ways to better incorporate sensor placement to improve aircraft manufacturing — not just what a sensor can measure and how it works, but where and how it should be affixed. Moths provide a great example of biological sensor placement; they have a series of biosensors within their wings that inform flight actions.
In complex neural systems, there are many biosensor patterns but only a few that dominate. My collaborators Bing Brunton, Nathan Kutz, Tom Daniel, Josh Proctor and I have created algorithms based on the moth’s sparse sensing. Tom got us interested in the moth, and Nathan introduced us to sparse sensing. Krithika Manohar, a former Ph.D. student of Nathan and mine, translated these algorithms to The Boeing Company to improve assembly rates on several aircraft lines.
Which industries are interested in data science?
Any industry that has lots of data! The tech and biomedical industries are at the forefront of this field. And the marketing and retail industries have long been key players. Manufacturing is increasing its investment in data science, too. Companies like Boeing and GE are leading efforts on this front.
For data to be valuable, it needs to be collected and analyzed. Companies that are making the biggest strides are those that are willing to invest in analytics. Over the years many have put resources toward data collection and storage, but few have invested in analyzing it. Data doesn’t do much good if there’s no means of interpreting and learning from it.
What advice do you have for ME students interested in data science?
Learn a programming language, become really good at math, and find a data project that you can delve into, apply your skills and get a sense of the research. It’s important to learn machine learning algorithms, but it’s just as important to dive in and experiment with data.
Keep up with Brunton and his team at eigensteve.com.
Originally published December 10, 2018