By Sarah DeWeerdt
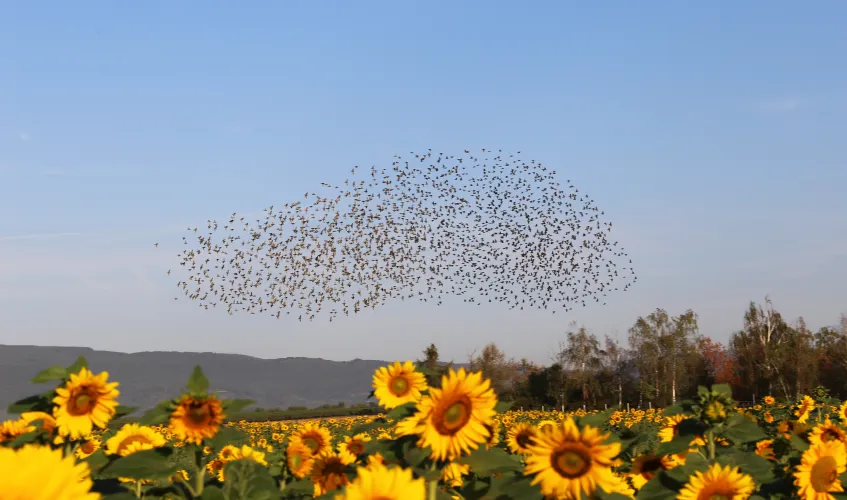
A flock of birds is an example of a distributed system in which individual members work together without central control.
Many of us have found ourselves transfixed by a flock of birds swooping and shifting direction in such perfect synchrony that the whole flock seems to shimmer in the air, a phenomenon sometimes known as a murmuration.
Engineers are fascinated by murmurations and flocking behavior too, albeit not so much for their beauty as for their practical import. A flock is an example of a distributed system — one in which individual members work together without central control.
Santosh Devasia
“As soon as something happens in one end, everyone knows what to do,” says mechanical engineering (ME) professor Santosh Devasia.
The development of autonomous robots — driverless cars, drones and so on — has touched off increasing interest in distributed systems. Distributed systems have many advantages — security, resilience, practicality. The question is how to make sure everyone knows what to do as seamlessly as happens in a flock of birds.
“How information propagates is very important,” Devasia says.
Devasia recently proposed a new mathematical model that captures this information propagation within flocks much more accurately than past models have been able to do. Now, he’s working to refine his model even further, and test whether it actually works when applied to robots in the real world.
Birds do it
Devasia got interested in murmurations about four years ago, when he heard a talk at a conference about research using data from real bird flocks to cast doubt on a standard mathematical model of flocking behavior that had prevailed for several decades.
The conventional model assumes that each bird looks to a set of neighbors to see what they are doing and adjusts its own flight path accordingly. The model’s equation is essentially the same as that used to explain heat transfer, such as how an incandescent light bulb warms the surrounding air.
In fact, that approach works pretty decently to describe the behavior of small flocks or ones moving slowly. But when the model is run with larger numbers or higher speeds, the flock splits apart — in contrast to the seamless unity of flocks in the real world.
“The model is very simple, the equation is really incredibly simple,” Devasia says. “And yet the birds have found a way to beat it.”
He wanted to find out the birds’ secret.
It turns out the problem is that in the conventional model, information transfer about rapid turn maneuvers dampens as it moves through the flock. (Similarly, if a light bulb is turned on for a little bit and then switched off, the transferred heat diminishes with distance: the air an inch from a light bulb is warmer than the air a foot away.)
Devasia’s model improves on the status quo by adding an additional mathematical term to the model equation. His version assumes that each bird adjusts what it’s doing based on its own past actions in addition to its observations of neighbors.
“So it’s an extra kick to what you’re trying to do,” Devasia says. He calls this concept ‘delayed self-reinforcement.’ In computer simulations using this new model, even large flocks moving and shifting directions rapidly remain perfectly synchronized, he reported in a paper published earlier this year.
Robot, take the wheel
But Devasia wasn’t content to just improve things. “As an engineer, the next question is, what’s the best I can do?” he laughs. In a forthcoming paper, he dives deeper into the rationale behind the new model and adds a few more mathematical refinements to further improve its performance.
These new refinements account for the fact that, unlike dots in a computer simulation, birds and robots can’t shift speed and direction instantly. “So the new theory allows us to then apply it to real-world problems,” Devasia says.
He’s beginning to do just that. In his lab, downstairs from his spare, tidy office in the Mechanical Engineering Building, Devasia’s team has built a fleet of 3-D printed robots that look like deconstructed toy cars. They want to see whether applying the new algorithm enables the cars to form tighter, more reliable groups, or ‘platoons,’ which saves both fuel and road space.
Another floor down, in the Boeing Advanced Research Center, which Devasia directs, he’s working on coordinating groups of robots to move larger structures. This could be useful in manufacturing processes, such as attaching a wing to an airplane.
If the approach pans out, it could have a host of other applications, such as coordinating robots in warehouses, fleets of drones delivering packages or arrays of satellites used in remote sensing.
Devasia even hopes that his model might aid in understanding the human brain. He’s been thinking about how neurons fire in a distributed manner, yet their activity adds up to organized patterns that underpin complex thought. Could the idea of delayed self-reinforcement yield insights into how this occurs?
And then there’s birdwatching. Devasia says he didn’t have any particular interest in birds before starting this line of research, but now he regularly finds himself captivated by the synchronicity of their movements.
“Unfortunately, that’s dangerous when you’re driving a car,” he says.
One more reason to get those autonomous vehicles operating smoothly.
Originally published November 18, 2019