By Lyra Fontaine
ME researchers improved a mathematical model describing how birds flock together – which could be applied to build robots that move in the same way.
ME researchers recently developed an improved mathematical model to describe how birds flock together while suppressing unwanted noise. Photo credit: Rhys Kentish
When the light turns green in an intersection, there’s a delay between when the driver in the first car sees the light change and moves forward, and when the driver in the eighth car in line moves forward. Even when the vehicles are autonomous or self-driving, the cars don’t start moving at the same time. More vehicles would be able to cross the intersection at the same light if they all “knew” that the light was turning green, started together and continued moving in the same way.
Traffic is one area studied by ME researchers, who recently came up with an improved mathematical model to describe how birds flock together while suppressing unwanted noise. This algorithm could be applied to build robots that work together better – whether they’re self-driving cars that can start driving at the same time, or small robots working in a large group to carry an item without stretching or breaking it.
“The applications are very diverse,” says ME Professor Santosh Devasia.
Devasia co-authored a paper, “Low-distortion information propagation with noise suppression in swarm networks,” recently published in Proceedings of the National Academy of Sciences. ME Professor Emeritus James Riley and ME alum Anuj Tiwari (Ph.D., ‘22) are also co-authors.
Inspired by nature
In swarms found in nature, information propagates without distortion. For example, to avoid predators, flocks of starling birds can perform parallel sharp turns. This flocking behavior is sometimes referred to as a murmuration. Devasia’s model takes into account that each bird adjusts its actions based on observations from its neighbors as well as its own previous actions, a concept he calls delayed self-reinforcement. He has since improved upon his own model.
Now, the model shows that delayed self-reinforcement can reduce distortion during information propagation, even in noisy environments. This new model could be used to improve cohesion in engineered networks, such as autonomous drone formations and in traffic.
The algorithm reflects how noise – such as the flapping noises of birds’ wings, or noise from cars moving – can be suppressed so that birds know where to fly next or the autonomous vehicle can “understand” its next action.
Related story
Distributed systems
The murmuration whisperer
ME professor Santosh Devasia is investigating how the flocking behavior of birds could inform autonomous robotics research.
“Our new method removes the high-frequency noise,” Devasia says. “You don’t want to follow the noise; you want to follow the motion.”
The model is a more accurate representation of how information is propagated in birds. Devasia became interested in the subject from learning about how flocks of starlings fly in gigantic swarms and maneuver together without centralized communication from a leader.
“Among birds, information doesn’t decay as it propagates,” Devasia says. “We’re trying to figure out why and change our mathematical models to reflect this behavior, because if you can do that, you can get autonomous vehicles and multiple robots to work together.”
The new model was also inspired by the dispersive properties of water waves, which are similar to how birds flock.
“It is often quite useful in engineering and mathematics to take advantage of how the results in one field of interest might directly apply to another, such as the cross-fertilization of one field with information from another,” says Riley, a fluid dynamicist who has studied wave dispersion extensively in problems of hydrodynamic stability, water waves and internal gravity waves.
Applying the algorithm
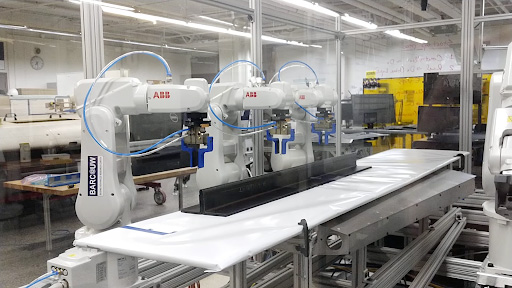
Devasia’s lab is applying the algorithm to develop reconfigurable robotic fixtures (shown above) that can work well together instead of traditional fixtures to hold and manipulate parts where each task requires a new specialized fixture. Instead, the same reconfigurable robotic system can handle a variety of parts and tasks.
Devasia’s team has done simulations with autonomous vehicles that demonstrated the advantages of using the new delayed self-reinforcement algorithm. The researchers found that more than triple the number of cars can move through the traffic light when compared to human drivers. Even with a 50-50 mix of autonomous vehicles and human-driven cars, there is a 30% increase in the number of cars that can get through the green light with the algorithm.
The lab is also interested in how multiple robots can be programmed to move at the same time so that they can carry large objects. In manufacturing, if a group of robots moves at different speeds or directions, it could damage the item they’re carrying.
Students in Devasia’s lab also built robots to carry large objects such as composite parts and applied the algorithm to them. They found only a small deviation between when the first and last robot moved.
Tiwari, who worked on the paper as a Ph.D. student, is grateful for his time in Devasia’s lab. This summer, he will become an assistant professor at IIT Gandhinagar India, where he plans to continue his research on networked systems and control.
“Studying the propagation of information through networks has not only enhanced my understanding of natural flocking behavior, but also allowed me to work in promising engineering applications for control of robotic and vehicular networks,” he says.
Devasia looks forward to applying the new algorithm to advanced manufacturing projects in the Boeing Advanced Research Center (BARC). He’s excited to continue to explore how one robot knows what the other is doing.
“How information propagates is really important,” he says.
Originally published April 10, 2023